Paper Collection
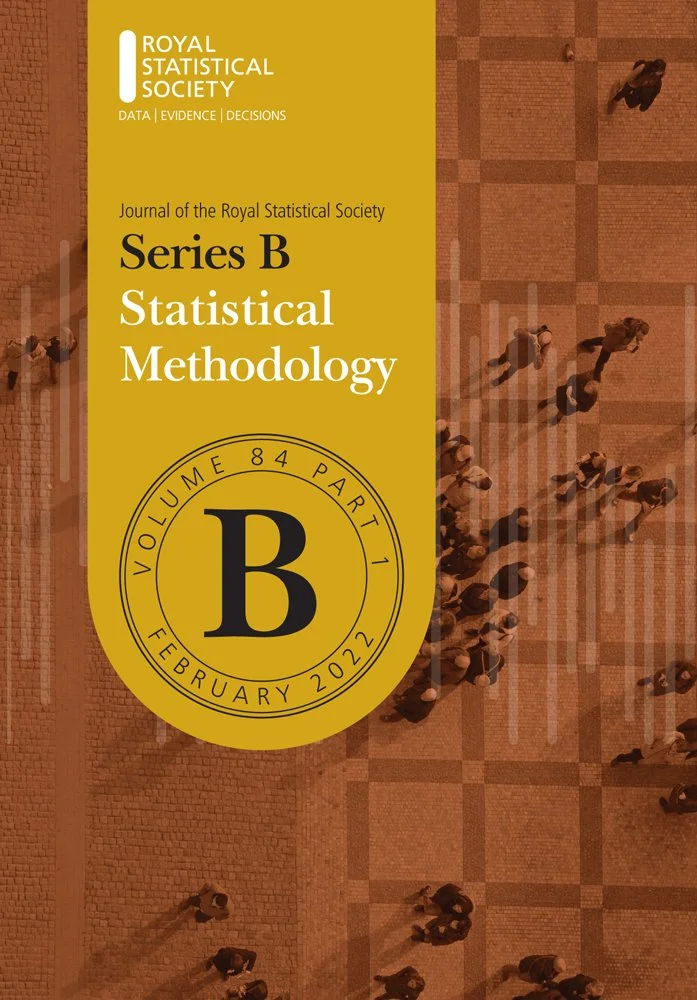
Robust Generalised Bayesian Inference for Intractable Likelihoods
Matsubara T, Knoblauch J, Briol FX, Oates CJ. Robust Generalised Bayesian Inference for Intractable Likelihoods. Journal of the Royal Statistical Society (Series B), 84(3):997-1022.
ISBA 2021 Best Student/Postdoc Contributed Paper Award
Best Student Paper Award, ASA Section on Bayesian Statistical Science, 2022
This work has been presented as a conference abstract at the NeurIPS 2021 Workshop “Your Model is Wrong: Robustness and Misspecification in Probabilistic Modeling”. [Web]

Optimal Thinning of MCMC Output
Riabiz M, Chen WY, Cockayne J, Swietach P, Niederer SA, Mackey L, Oates CJ. Optimal Thinning of MCMC Output. Journal of the Royal Statistical Society (Series B), 84(4):1059-1081.
[Journal] [arXiv] [Software] [Blog1] [Blog2] [Video]
This work has been presented as a conference abstract at the Third Symposium on Advances in Approximate Bayesian Inference (AABI 2020). [Web] [Video]
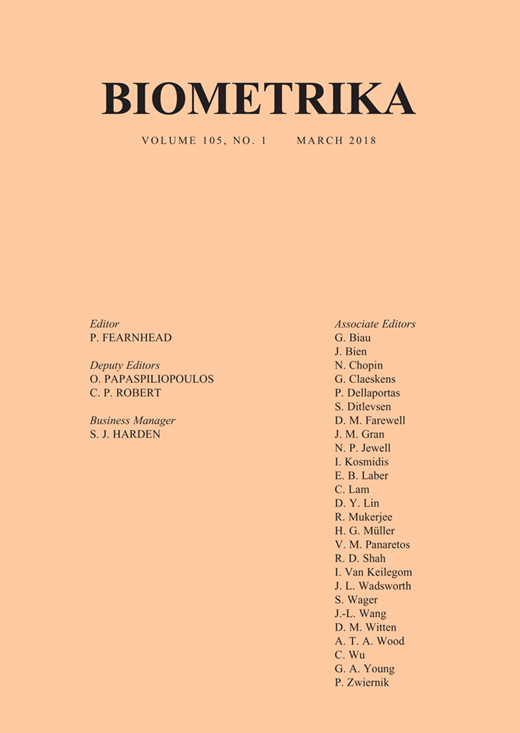
Semi-Exact Control Functionals From Sard's Method
South LF, Karvonen T, Nemeth C, Girolami M, Oates CJ. Semi-Exact Control Functionals From Sard's Method. Biometrika, 109(2):351–367.

Scalable Control Variates for Monte Carlo Methods via Stochastic Optimization
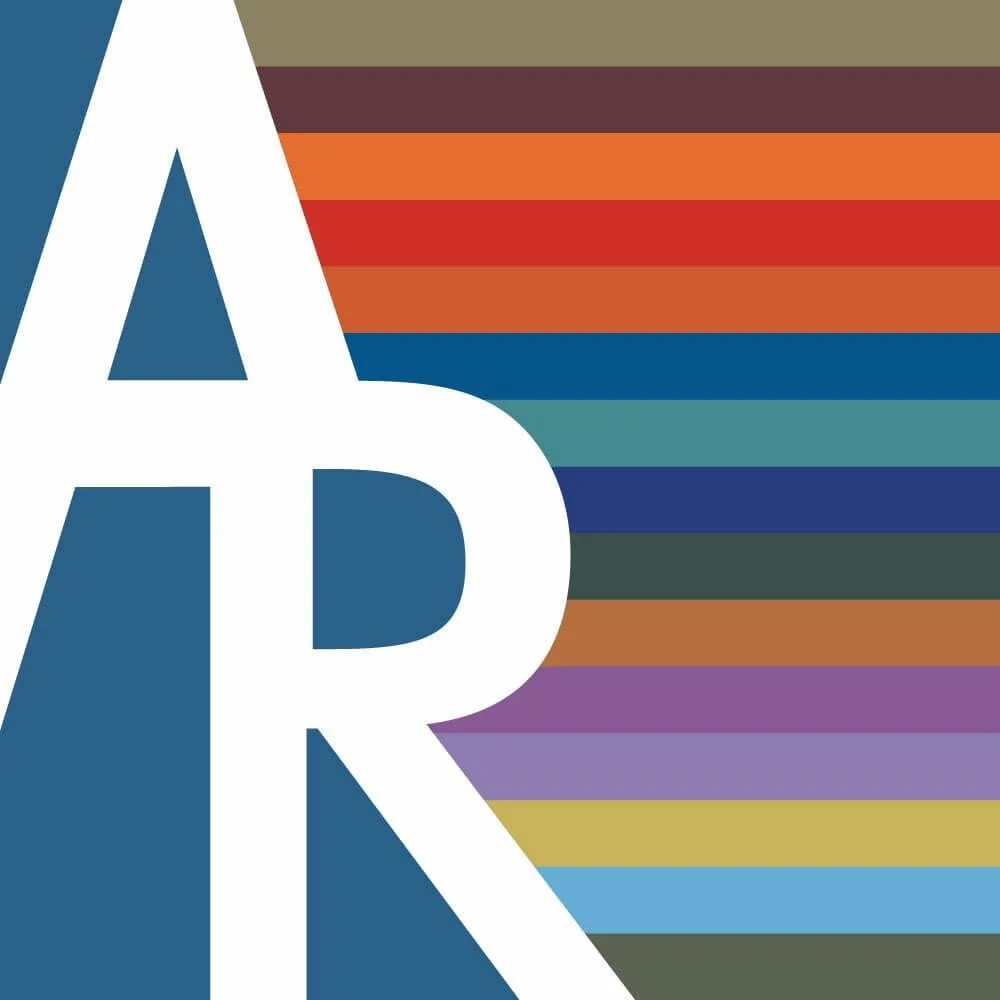
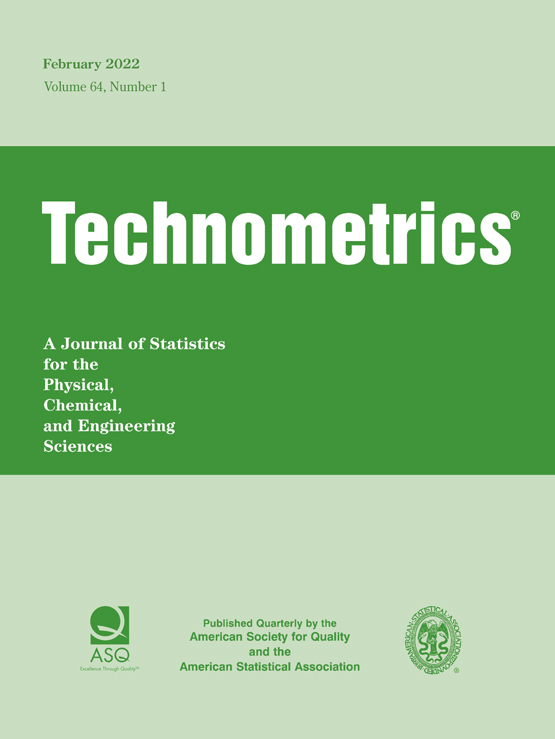
A Statistical Approach to Surface Metrology for 3D-Printed Stainless Steel
Oates CJ, Kendall WS, Fleming L. A Statistical Approach to Surface Metrology for 3D-Printed Stainless Steel. Technometrics, 64(3):370-383.
This work has been presented as a conference abstract: Oates CJ, Kendall WS, Fleming L. Generative Modelling of Rough Surfaces: An Application to 3D-Printed Stainless Steel. NeurIPS 2020 Workshop on Machine Learning for Engineering Modeling, Simulation, and Design. [Web]


A Data-Centric Approach to Generative Modelling for 3D-Printed Steel
Dodwell TJ, Fleming LR, Buchanan C, Kyvelou P, Detommaso G, Gosling PD, Scheichl R, Kendall WS, Gardner L, Girolami MA, Oates CJ. A Data-Centric Approach to Generative Modelling for 3D-Printed Steel. Proceedings of the Royal Society A, 477(2255).
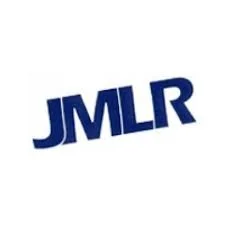


The Ridgelet Prior: A Covariance Function Approach to Prior Specification for Bayesian Neural Networks
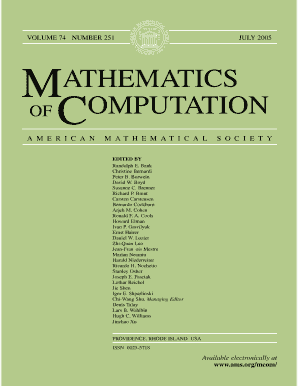

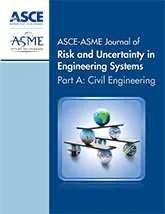
Causal Graphical Models for Systems-Level Engineering Assessment
Stephenson V, Oates CJ, Finlayson A, Thomas C, Wilson K. Causal Graphical Models for Systems-Level Engineering Assessment. ASCE-ASME Journal of Risk and Uncertainty in Engineering Systems, Part A: Civil Engineering, 7(2):04021011.


Maximum Likelihood Estimation and Uncertainty Quantification for Gaussian Process Approximation of Deterministic Functions

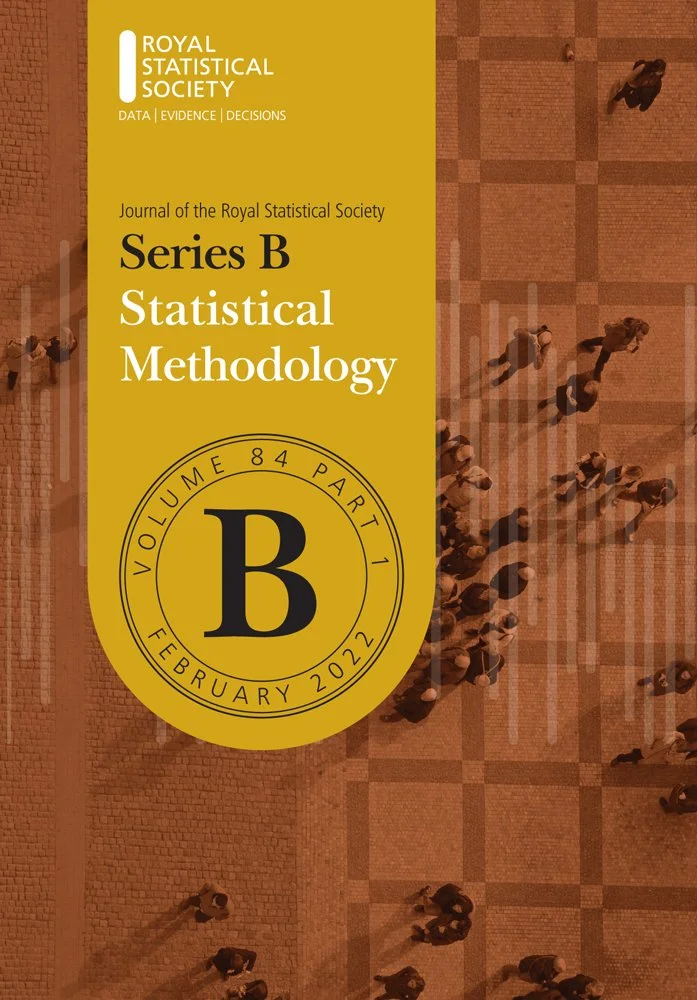
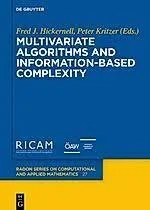
Optimality Criteria for Probabilistic Numerical Methods
Oates CJ, Cockayne J, Prangle D, Sullivan TJ, Girolami M. Optimality Criteria for Probabilistic Numerical Methods. In Multivariate Algorithms and Information-Based Complexity, eds, Hickernell, Kritzer, Berlin/Boston De Gruyter.


Editorial: Special Edition on Probabilistic Numerics
Girolami M, Ipsen I, Oates CJ, Owen A, Sullivan T. Editorial: Special Edition on Probabilistic Numerics. Statistics and Computing, 29(6):1181-1183.

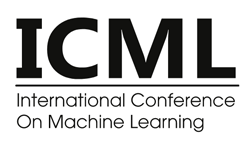
Stein Point Markov Chain Monte Carlo
Chen WY, Barp A, Briol FX, Gorham J, Girolami M, Mackey L, Oates CJ. Stein Point Markov Chain Monte Carlo. International Conference on Machine Learning (ICML 2019).


Bayesian Probabilistic Numerical Methods
Cockayne J, Oates CJ, Sullivan T, Girolami M. Bayesian Probabilistic Numerical Methods. SIAM Review, 61(4):756-789.
[Journal] [arXiv] [Video1] [Video2] [Video3] [Blog]
Best Student Paper Prize, ASA Section on Bayesian Statistical Science

Symmetry Exploits for Bayesian Cubature Methods
Karvonen T, Särkkä S, Oates CJ. Symmetry Exploits for Bayesian Cubature Methods. Statistics and Computing, 29:1231-1248.

A Bayesian Conjugate Gradient Method
Cockayne J, Oates CJ, Ipsen I, Girolami M. A Bayesian Conjugate Gradient Method (with discussion and rejoinder). Bayesian Analysis, 14(3):937-1012.
[Journal] [arXiv] [Software] [Webinar]
This was the first ever discussion paper webinar held by the journal.
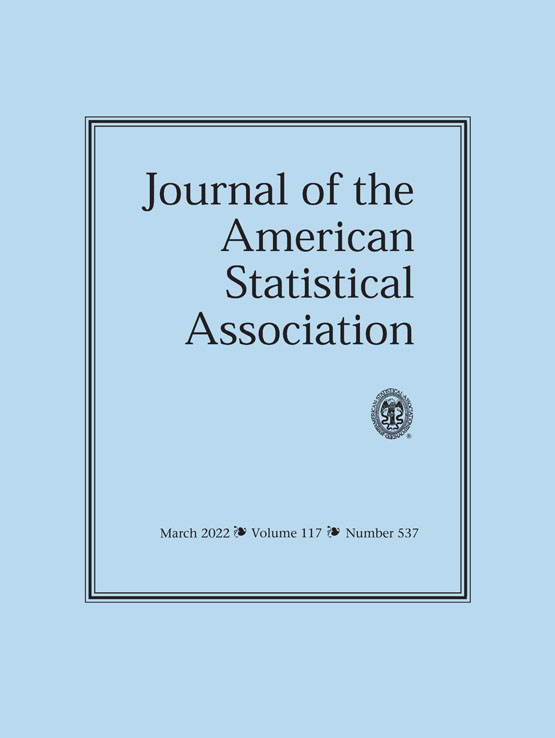
Bayesian Probabilistic Numerical Methods in Time-Dependent State Estimation for Industrial Hydrocyclone Equipment
Oates CJ, Cockayne J, Aykroyd RG, Girolami M. Bayesian Probabilistic Numerical Methods in Time-Dependent State Estimation for Industrial Hydrocyclone Equipment. Journal of the American Statistical Association, 114(528):1518-1531.

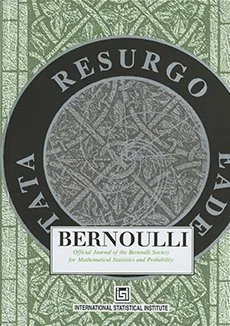
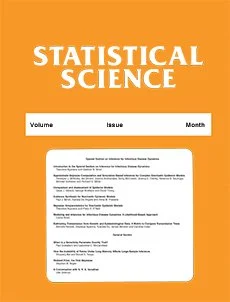
Probabilistic Integration: A Role in Statistical Computation? (with discussion and rejoinder)
Briol F-X, Oates, CJ, Girolami, M, Osborne, MA, Sejdinovic, D. Probabilistic Integration: A Role in Statistical Computation? (with discussion and rejoinder) Statistical Science, 34(1):1-22. (Rejoinder on p38-42.)
[Journal] [Discussion1] [Discussion2] [Discussion3] [Rejoinder] [arXiv] [Video] [Poster] [Blog1] [Blog2] [Blog3] [Blog4] [Blog5] [ProbNum]
Best Student Paper Prize, ASA Section on Bayesian Statistical Science

Graphical Models in Molecular Systems Biology
Mukherjee S, Oates CJ. Graphical Models in Molecular Systems Biology. In Handbook of Graphical Models, eds. Maathuis M, Drton M, Lauritzen S, Wainwright M, CRC Press.

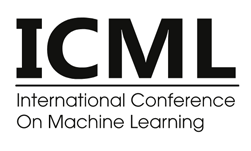

Probabilistic Models for Integration Error in Assessment of Functional Cardiac Models

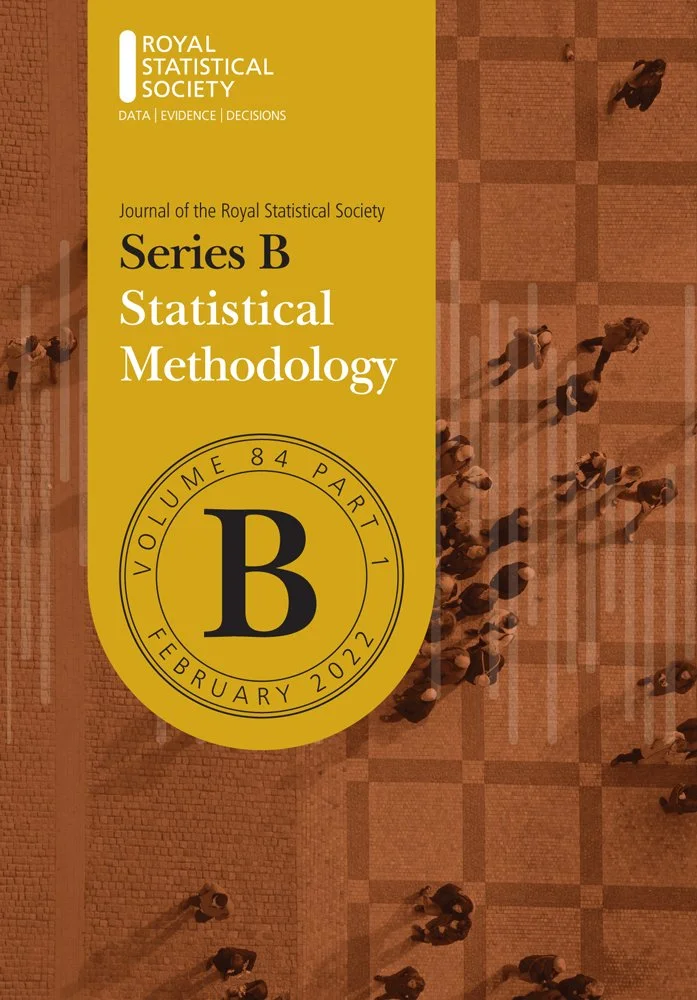
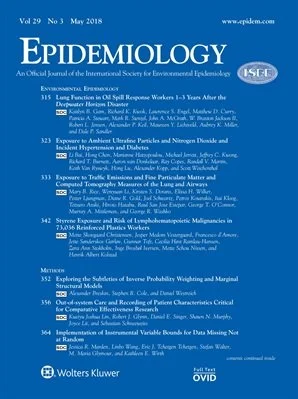
Repair of Partly Misspecified Causal Diagrams
Oates CJ, Kasza J, Simpson JA, Forbes AB. (2017) Repair of Partly Misspecified Causal Diagrams. Epidemiology, 28(4):548-552.
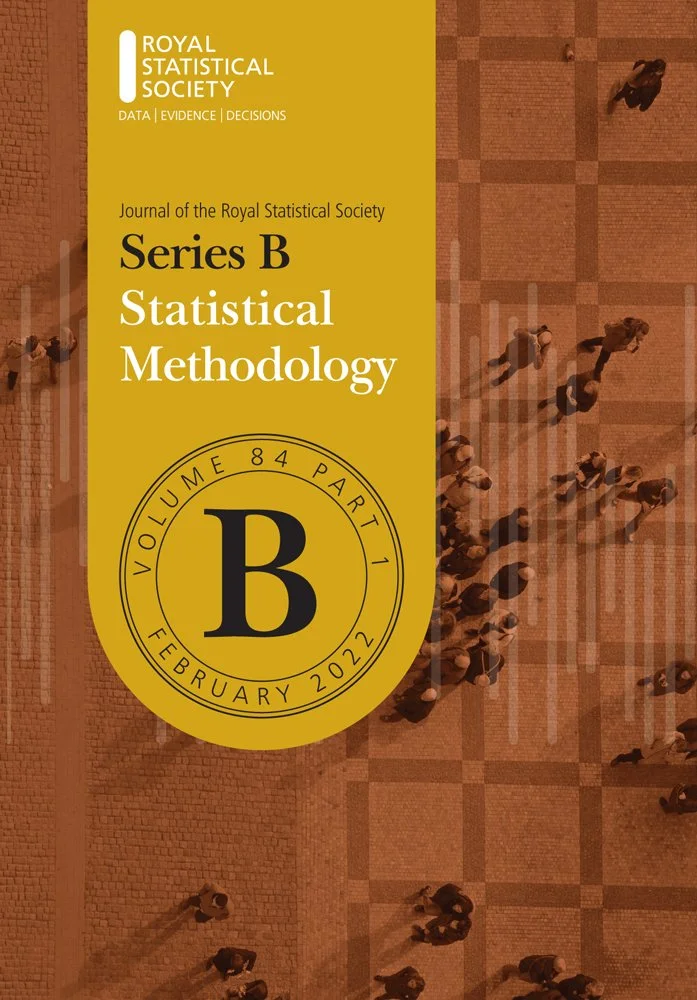
Control Functionals for Monte Carlo Integration
Oates CJ, Girolami M, Chopin N. (2017) Control Functionals for Monte Carlo Integration. Journal of the Royal Statistical Society, Series B, 79(3):695-718.


Probabilistic Numerical Methods for PDE-constrained Bayesian Inverse Problems
Cockayne J, Oates CJ, Sullivan T, Girolami M (2016) Probabilistic Numerical Methods for PDE-constrained Bayesian Inverse Problems. Proceedings of the 36th International Workshop on Bayesian Inference and Maximum Entropy Methods in Science and Engineering, Ed. Geert Verdoolaege, AIP Conference Proceedings, 1853:060001.
This is a short form of the full paper: Cockayne J, Oates CJ, Sullivan T, Girolami M. Probabilistic Meshless Methods for Bayesian Inverse Problems.

Discussion of “Causal inference using invariant prediction: identification and confidence intervals”

RNA editing generates sequence diversity within cell populations
Harjanto D, Papamarkou T, Oates CJ, Rayon Estrada V, Papavasiliou FN, Papavasiliou A. (2016) RNA editing generates sequence diversity within cell populations. Nature Communications, 7:12145.
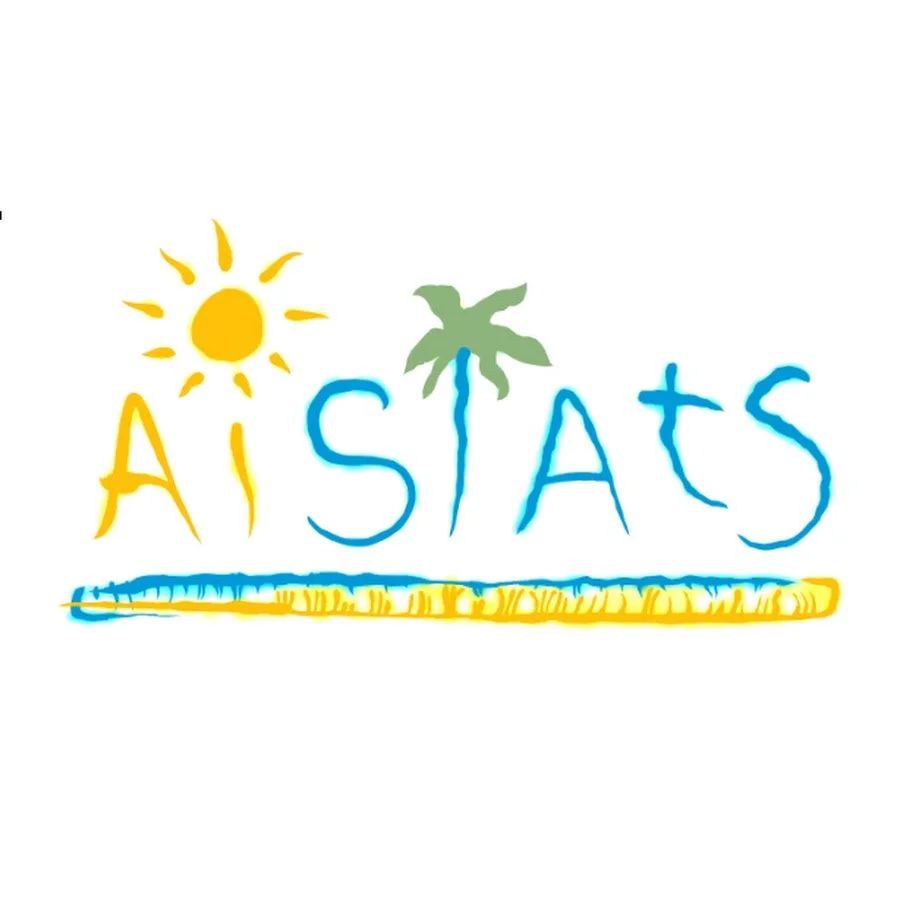
Control Functionals for Quasi-Monte Carlo Integration
Oates CJ, Girolami M. (2016) Control Functionals for Quasi-Monte Carlo Integration. Nineteenth International Conference on Artificial Intelligence and Statistics (AISTATS), Journal of Machine Learning Research W&CP, 51:56-65.
Selected for Oral Presentation (top 6.5% of submissions)
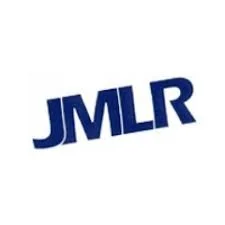
Estimation of Causal Structure Using Conditional DAG Models
Oates CJ, Smith JQ, Mukherjee S. (2016) Estimation of Causal Structure Using Conditional DAG Models. Journal of Machine Learning Research, 17(54):1−23.

The Controlled Thermodynamic Integral for Bayesian Model Evidence Evaluation


Exact Estimation of Multiple Directed Acyclic Graphs
Oates CJ, Smith JQ, Mukherjee S, Cussens J (2016) Exact Estimation of Multiple Directed Acyclic Graphs. Statistics and Computing, 26(4):797-811.

Frank-Wolfe Bayesian Quadrature: Probabilistic Integration with Theoretical Guarantees

Decoupling of the PI3K pathway via mutation necessitates combinatorial treatment in HER2+ breast cancer
Korkola JE, Collisson EA, Heiser L, Oates CJ, Bayani N, Itani, S, Esch, A, Thompson, W, Griffith OL,Wang NJ, Kuo W-L, Cooper B, Billig J, Ziyad S, Hung JL, Jakkula L, Lu Y, Mills G, Spellman PT, Tomlin, C., Mukherjee S, Gray JW. (2015) Decoupling of the PI3K pathway via mutation necessitates combinatorial treatment in HER2+ breast cancer. PLoS One, 10(7):e0133219.
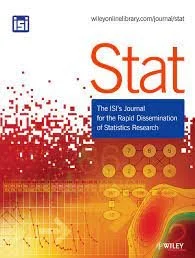
Accelerated Nonparametrics for Cascades of Poisson Processes
Oates CJ. (2015) Accelerated Nonparametrics for Cascades of Poisson Processes. Stat, 4(1):183-195.
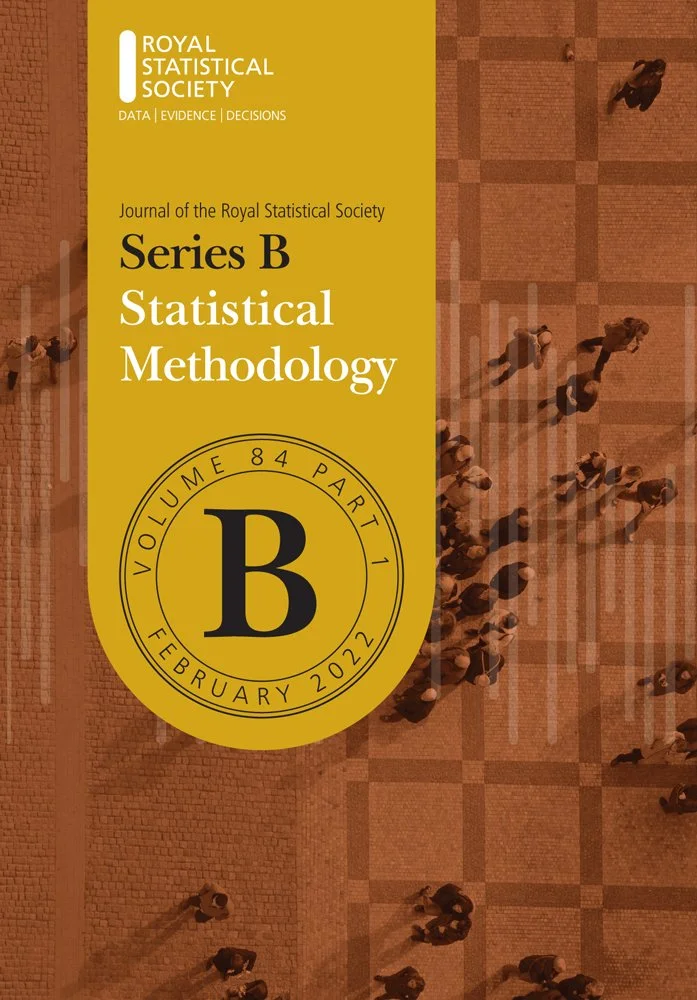
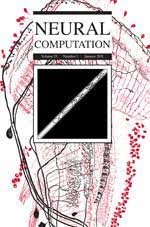
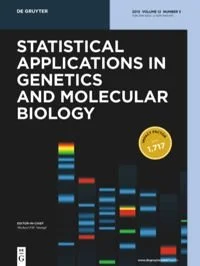
Quantifying the Multi-Scale Performance of Network Inference Algorithms
Oates CJ, Amos R, Spencer SEF (2014) Quantifying the Multi-Scale Performance of Network Inference Algorithms. Statistical Applications in Genetics and Molecular Biology 13(5):611-631.

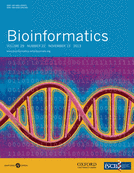
Causal network inference using biochemical kinetics
Oates CJ, Dondelinger F, Bayani N, Korkola J, Gray JW, Mukherjee S (2014) Causal network inference using biochemical kinetics. Bioinformatics 30(17):i468-i474.
Best Paper at the European Conference on Computational Biology 2014

Joint Structure Learning of Multiple Non-Exchangeable Networks

Single-Cell States in the Estrogen Response of Breast Cancer Cell Lines
Casale FP, Giurato G, Nassa G, Armond J, Oates CJ, Corà D, Gamba A, Mukherjee S, Weisz A, Nicodemi M (2014) Single-Cell States in the Estrogen Response of Breast Cancer Cell Lines. PLoS One 9(2):e88485.

A stochastic model dissects cellular states and heterogeneity in transition processes

Bayesian Inference for Protein Signalling Networks
Oates CJ (2013) Bayesian Inference for Protein Signalling Networks. PhD Thesis.

Self Organisation and Emergence
Chau Y-X, Oates CJ, Rana AA, Robinson L, Nicodemi M. (2013) Self Organisation and Emergence. In: Complexity Science: The Warwick Master’s Course (London Mathematical Society Lecture Note Series). Ed. by Ball R, Kolokoltsov V, MacKay R., Cambridge University Press.

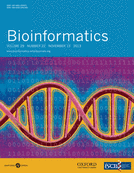

Measure Transport with Kernel Stein Discrepancy
Fisher MA, Nolan T, Graham MM, Prangle D, Oates CJ. Measure Transport with Kernel Stein Discrepancy, AISTATS 2021.
Selected or oral presentation (top 3%)
(Note that the arXiv version corrects errors in the AISTATS version.)
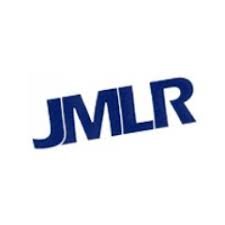
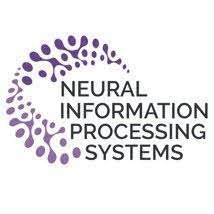
Gradient-Free Kernel Stein Discrepancy
Fisher M, Oates CJ. Gradient-Free Kernel Stein Discrepancy. Advances in Neural Information Processing Systems (NeurIPS 2023) [arXiv]
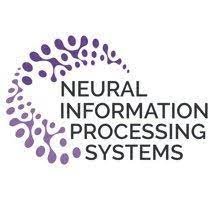
Stein Π-Importance Sampling
Wang C, Chen WY, Kanagawa H, Oates CJ. Stein Π-Importance Sampling. Advances in Neural Information Processing Systems (NeurIPS 2023) [arXiv]
Selected for spotlight presentation.
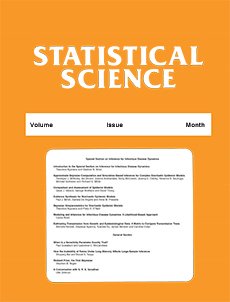
Stein's Method Meets Statistics: A Review of Some Recent Developments
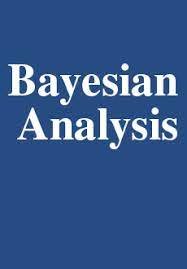

Parameter Space Reduction for Four-chamber Electromechanics Simulations Using Gaussian Processes Emulators
Strocchi M, Longobardi S, Augustin CM, Gsell MAF, Vigmond EJ, Plank G, Oates CJ, Wilkinson RD, Niederer SA. Parameter Space Reduction for Four-chamber Electromechanics Simulations Using Gaussian Processes Emulators. In Proceedings of the 10th Vienna International Conference on Mathematical Modelling, 2022.

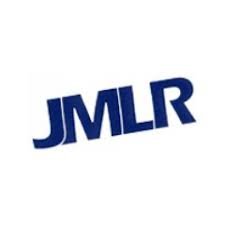
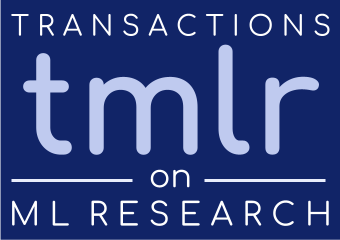
Sobolev Spaces, Kernels and Discrepancies over Hyperspheres
Hubbert S, Porcu E, Oates CJ, Girolami M (2023) Sobolev Spaces, Kernels and Discrepancies over Hyperspheres. Transactions on Machine Learning Research.

Meta-learning Control Variates: Variance Reduction with Limited Data
Sun Z, Oates CJ, Briol FX. Meta-learning Control Variates: Variance Reduction with Limited Data. Conference on Uncertainty in Artificial Intelligence (UAI 2023)
Selected for oral presentation.
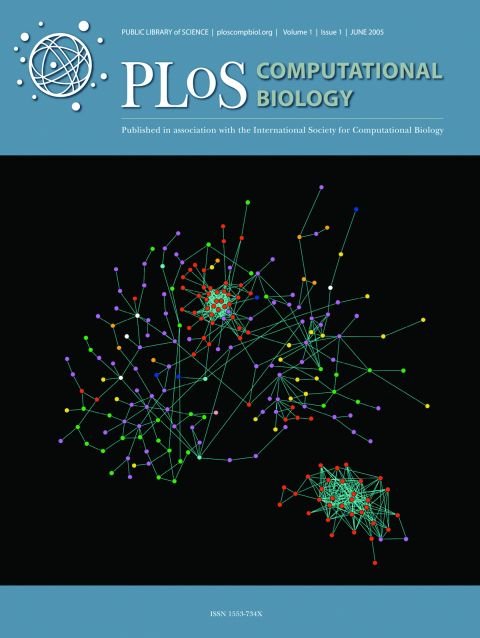
Cell to Whole Organ Global Sensitivity Analysis on a Four-chamber Electromechanics Model Using Gaussian Processes Emulators
Strocchi M, Longobardi S, Augustin CM, Gsell MAF, Petras A, Rinaldi CA, Vigmond EJ, Plank G, Oates CJ, Wilkinson RD, Niederer SA. Cell to Whole Organ Global Sensitivity Analysis on a Four-chamber Electromechanics Model Using Gaussian Processes Emulators. PLoS Computational Biology, 19(6): e1011257. [Journal]
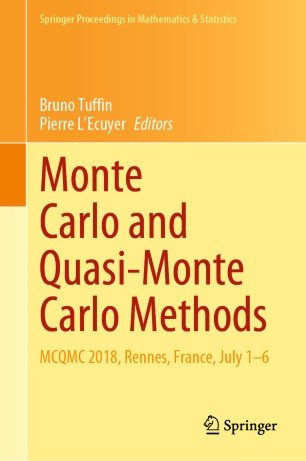

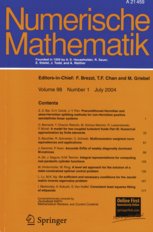

Review of "Probabilistic Numerics" by Hennig, Osborne and Kersting
Oates CJ. Review of "Probabilistic Numerics" by Hennig, Osborne and Kersting. SIAM Review, 65(3):905-915. [Journal]
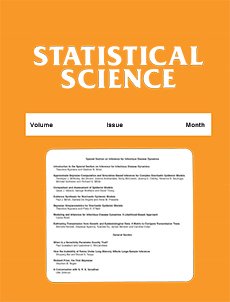
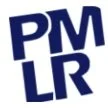
GaussED: A Probabilistic Programming Language for Sequential Experimental Design
Fisher MA, Teymur O, Oates CJ. GaussED: A Python Package for Sequential Experimental Design. Proceedings of the First International Conference on Probabilistic Numerics, 2025. [arXiv]
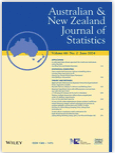
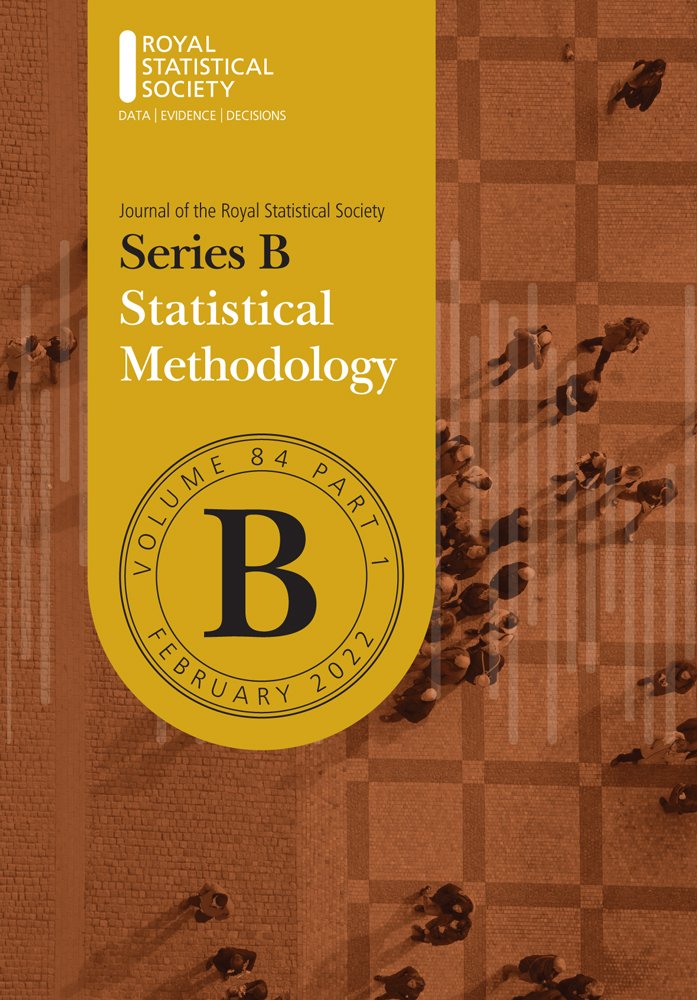
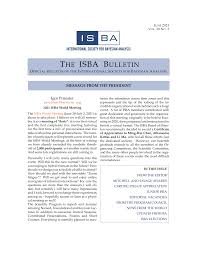
Grand Challenges in Bayesian Computation
Bhattacharya A, Linero A, Oates CJ (2024) Grand Challenges in Bayesian Computation. ISBA Bulletin 31(3). [arXiv]
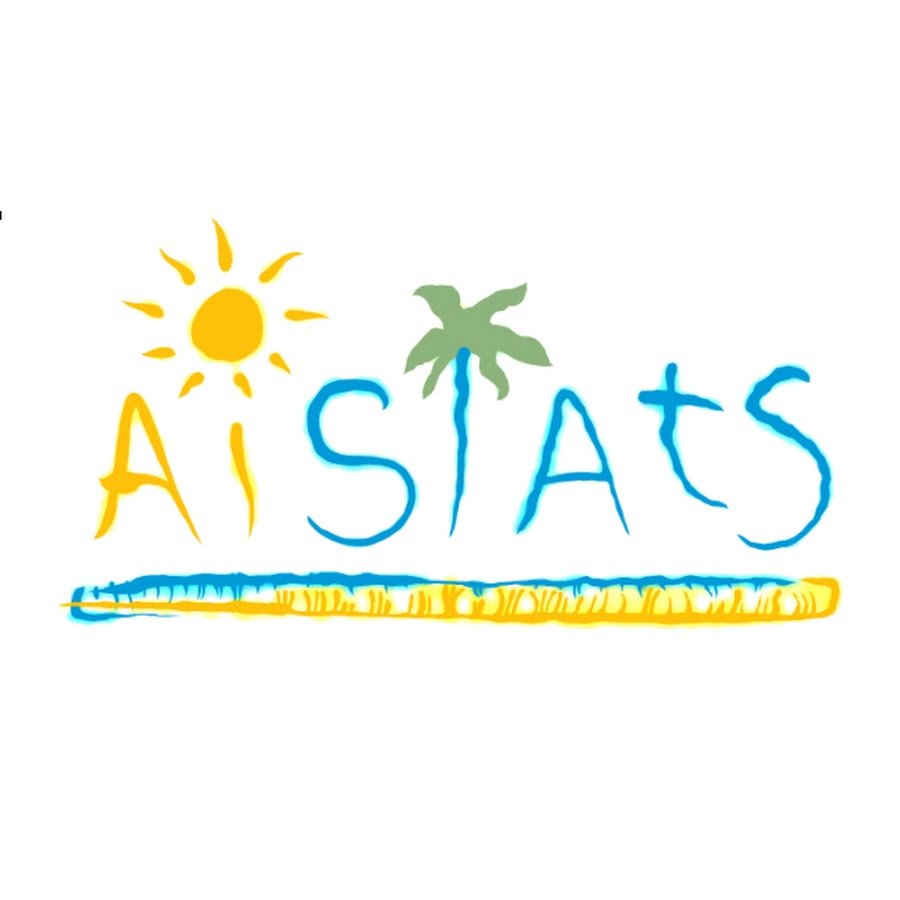
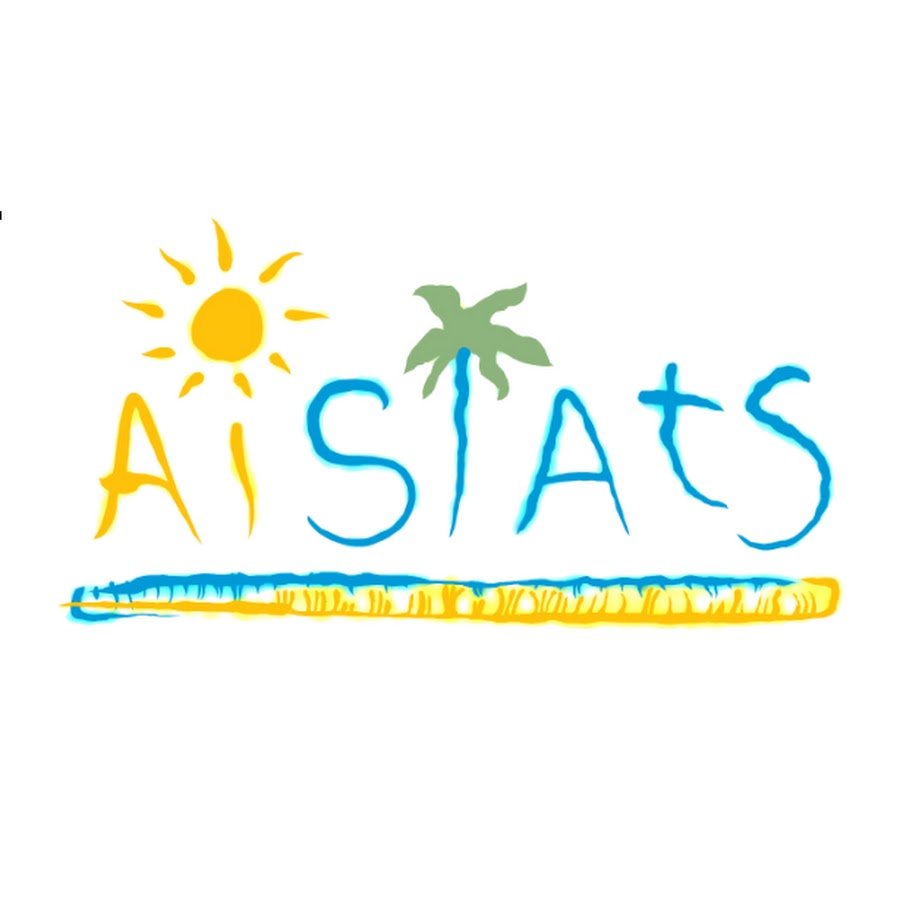
Reinforcement Learning for Adaptive MCMC
Wang C, Chen W, Kanagawa H, Oates CJ. Reinforcement Learning for Adaptive MCMC. Artificial Intelligence and Statistics (AISTATS 2025) [arXiv]
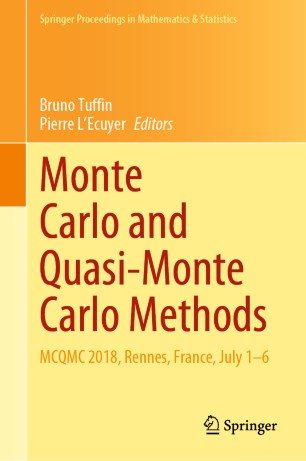
Fast Approximate Solutions of Stein Equations for Post-Processing of MCMC
Liu Q, Kanagawa H, Fisher MA, Briol F-X, Oates CJ. Fast Approximate Solutions of Stein Equations for Post-Processing of MCMC. To appear in: Lemieux C, Feng B (eds.). Monte Carlo and Quasi-Monte Carlo Methods 2024. Springer Verlag. [arXiv]